Surpassing Frontier AI for CPG & Retail: Tickr AI beats OpenAI & Anthropic at Dynamic Hierarchical Product Categorization
Dynamic Hierarchical Product Categorization (DHPC) in CPG & Retail
In the consumer packaged goods (CPG) industry, where an average of 30,000 new products are launched each year—a well-structured product hierarchy is crucial. This enhances the customer experience by making it easier and quicker to find products, whether online or in physical stores. This ease of navigation can lead to increased sales, store traffic, and larger basket sizes. Effective product categorization also empowers marketers and retailers to better understand customer segments, tailor marketing plans, and analyze trends impacting inventories and pricing strategies. By leveraging dynamic hierarchical product categorization, businesses can identify gaps in their offerings, seize opportunities for new products, and optimize their product portfolios.
Tickr’s DHPC streamlines and automates the process across any category set, significantly reducing costs and saving time. Our AI system delivers unmatched efficiency, outperforming human speed by 100-1,000x. Designed for seamless integration, Tickr’s solution adapts effortlessly to existing systems, regardless of category structures. It can instantly identify new items, categorizing them accurately or matching them to existing products, eliminating the need for costly retraining or manual updates. This adaptability allows businesses to focus on growth without the burden of ongoing maintenance.
DHPC is a comprehensive AI system, encompassing search, preprocessing and post-processing pipelines, an inference stack, and more. At its core are our large language models: our base model Tickr-LLM-base and fine-tuned Tickr-LLM-fine-tuned deployments. Tickr’s platform is also fully interoperable, seamlessly integrating with frontier models from OpenAI and Anthropic, ensuring that businesses can achieve high accuracy and efficiency in managing dynamic product categories, all while being tailored to their specific business and security compliance needs.
Our models, including Tickr-LLM-base and Tickr-LLM-fine-tuned, demonstrate exceptional performance, surpassing leading frontier models from OpenAI and Anthropic. As shown in the Figure 1, Tickr-LLM-base achieves a strong baseline accuracy of 90.8%, and when fine-tuned, the model reaches an impressive 98.1% accuracy—far outperforming competing models such as GPT-4o, GPT-4o-mini, Claude Sonnet 3.5, which hover around 86%. This performance advantage is driven by our models’ superior ability to attend to few-shot examples without category descriptions, learning quickly from demonstrations without requiring extensive retraining.
Why Generative?
Traditional discriminative models classify inputs by mapping them to a fixed set of predefined categories. While effective for stable tasks, they lack flexibility when faced with dynamic or expanding category spaces—a common scenario in CPG and retail, where product categories frequently evolve, grow, and originate from various data sources. Discriminative models require retraining to accommodate new classes, making them inefficient for managing thousands of changing categories.
In contrast, generative models like modern LLMs offer superior adaptability and advanced reasoning capabilities for tasks like Hierarchical Product Categorization. Unlike smaller, pre-trained encoder-only models such as BERT-large, which are constrained by predefined classes and rely on retraining for new categories, LLMs are significantly larger and possess a level of “reasoning” that encoder-only models currently lack. This reasoning emerges from large-scale pretraining across exhaustive web-scale data, resulting in a world model that leverages additional context. LLMs can interpret and generate hierarchical categorizations based on context and demonstrations (in-context learning), allowing them to adapt to new or uniquely defined categories on the fly. This flexibility provides the scalability needed to handle complex, changing categories, leading to more accurate and efficient solutions.
Benchmarking Against the Frontier
We compare Tickr-LLM-base and Tickr-LLM-fine-tuned against are GPT-4o, GPT-4o-mini, and Claude Sonnet 3.5. We omit OpenAI’s Strawberry and Anthropic’s Opus models as they do not scale in cost or time saved for any of the large-scale process automation tasks Tickr solves, like Hierarchical Product Categorization.
We focus specifically on the ability of the models to use demonstrations without any explanations of the categories. This is important because some of our clients have thousands of categories and each client’s categorization structure is unique Thus the ability to learn from examples is important for using LLMs for Hierarchical Product Categorization (and other tasks in retail and CPG).
Our Methodology
To thoroughly evaluate the models, we used four datasets with different categories and hierarchies. This amounts to hundreds of categories and thousands of subcategories. Each dataset presents unique challenges and levels of granularity, simulating the diverse and complex classification tasks our clients face daily. The data is messy and simulates the real world. This includes different categories mapping to the same product or ambiguous category decision boundaries. Tickr has been given permission to run these experiments and we strive to protect our partners’ and clients’ data, so we will not publish or provide additional information about the data source.
Each model, including our own, is sent the exact same instructions (prompt). We include a template from the prompt below:
The taxonomic classification of a product is defined as a hierarchy of categories, where each category is a class. Because we are dealing with arbitrary datasets, the classes are not standardized and can be anything from a simple category to a complex taxonomy. Use the demonstrations, your knowledge of products, and your knowledge of the world to predict the products hierarchical categorization.
{demonstrations}
Tickr-LLM-base is pre-trained on nearly one million retrieval-augmented instructions & Tickr-LLM-fine-tuned represents four different Quantized Low Rank Adapters (QLoRAs) across the four datasets used for performance benchmarking. QLoRAs are a fine-tuning technique that compresses large language models by approximating their weights with low-rank matrices, significantly reducing computational costs and memory usage while maintaining high performance on new tasks. Demonstrations are found via our AI search algorithms. Their quality and the number of demonstrations effect downstream performance.
Super-frontier Performance
The high accuracy of our models demonstrate their ability to use demonstrations without additional context, as this important for the dynamism of product categorization.
Tickr AI LLMs lead with a 90.8% accuracy rate for Tickr-LLM-base & 98.1% for Tickr-LLM-fine-tuned. Claude Sonnet 3.5 follows with an accuracy of 86.6%, and GPT-4o and GPT-4o-mini both achieve 86.2% & 86.1%. While all models demonstrate competitive performance, Tickr LLMs consistently achieves higher accuracy across the datasets. We note that the near equivalent performance of GPT-4o & GPT-4o-mini is likely due to GPT-4o-mini being distilled from GPT-4o.
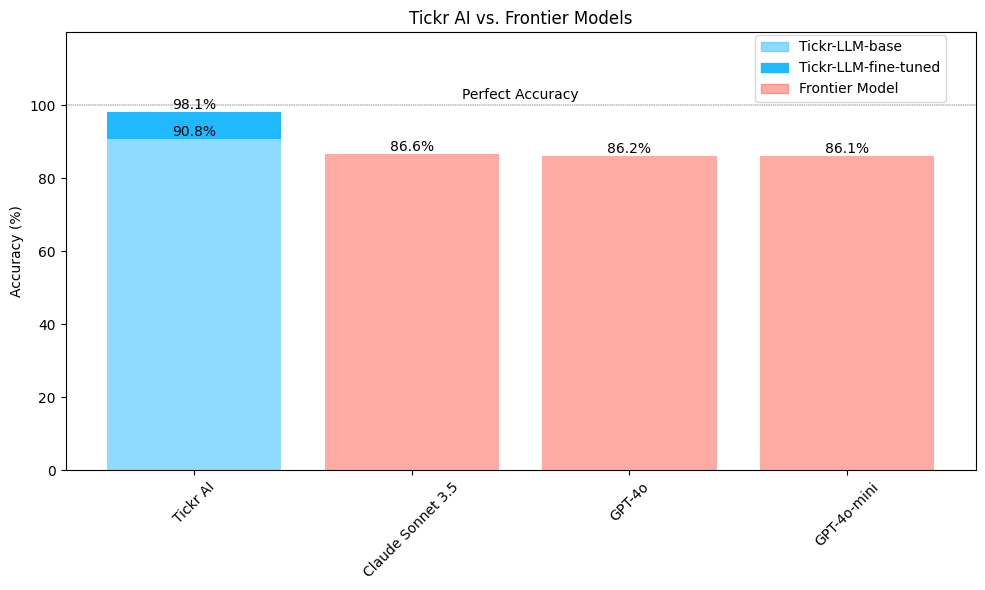
Closing Remarks
At Tickr AI, we strive to help our clients automate their largest inefficiencies and organizational challenges with AI, ultimately leading to significant cost and time savings. We developed Tickr-LLM-base and Tickr-LLM-finetuned to deliver state-of-the-art performance for dynamic hierarchical product categorization. These models are designed to adapt to the complexities of evolving product categories, offering reliable, scalable solutions that reduce manual effort and improve speed-to-market for CPG and retail businesses.
For more information on how Tickr AI can optimize your business, contact us at info@tickr.com.
- Publish Date
- October 23rd, 2024
- Abstract
- Tickr's Dynamic Hierarchical Product Categorization offers CPGs and retailers a scalable, AI-powered solution that automates the task of categorizing products across evolving categories, significantly reducing manual effort and operational costs. Utilizing Tickr-LLM-base and Tickr-LLM-fine-tuned, the system achieves up to 98.1% accuracy, outperforming leading models like GPT-4o and Claude Sonnet 3.5. This superior performance enables businesses to streamline processes, enhance efficiency, improve downstream data science applications and reducing time spent on maintenance.